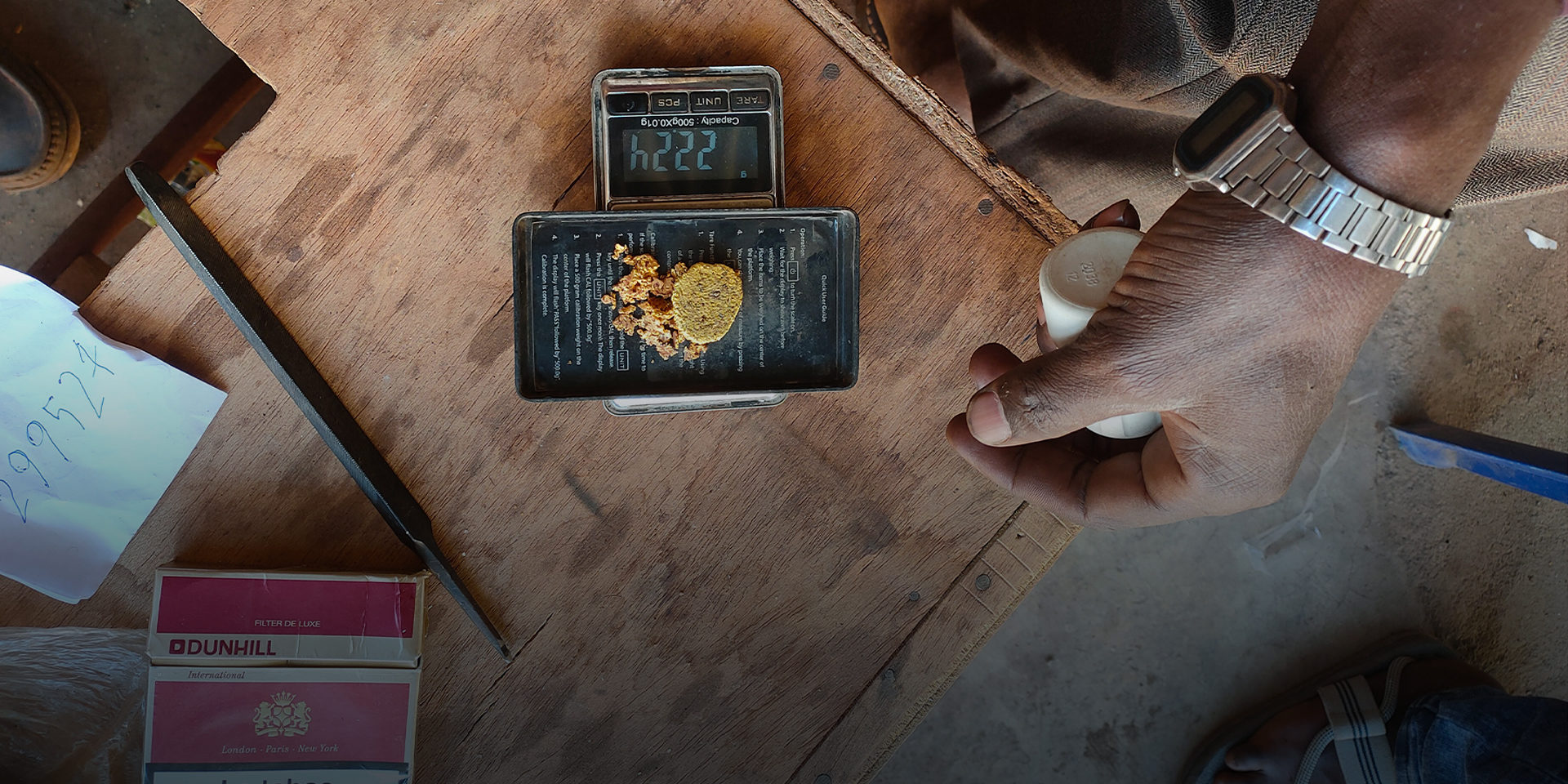
Let’s Get Creative: Localized Data Collection for Stronger Project Outcomes
December 23, 2019 | 4 Minute ReadHow can the development community become more creative with data collection to navigate complex operating environments and gather accurate, high-quality, consistent data?
In discussing and measuring project success, the international development community is increasingly turning to “data-driven deliverables” that collect solid, demonstrable data to show project results and to build, design, and implement successful development programs. Yet the development industry, by nature, works in places where high-quality, reliable, and consistent data is hard to collect. Local environments, conflicts, and capacity challenges often make data in the development industry unstable and inconsistent, which in turn can lead implementers to misjudged conclusions and failed outcomes. For this reason, the development community must think creatively about how we collect data and analyze successes, while understanding that no model is a one-size-fits all. Tailoring data collection methods for local contexts and challenges is in turn necessary to adjust for the unpredictability of development settings. “Localized” in this context refers to a data collection model built from the ground up to best suit the needs of a project, its location, and its resources. Creative, localized data collection can help to more clearly demonstrate results and to convert statistics into actionable information for the client and the project.
Thinking creatively about what data means, what it can look like, and how it can be verified is critical in making the transition to technical and data-driven outputs successful. One recent example of creative data collection is the Security Sector Governance (SSG) project’s survey of small-scale gold mines in Mali. Mali can be a dangerous operating environment, replete with violent extremist groups, criminal organizations, and trafficking of weapons, gold, goods, people, and drugs. Furthermore, these dangerous actors often operate in and around small gold mines, as gold can easily be used for money laundering. The sensitive nature of the environment and the data necessitated a localized, creatively designed data collection mechanism to minimize the inherent liabilities of the terrain and to retrieve the best information possible.
For this survey, the SSG project implemented three different data collection models to suit varying situations across the mining industry. The primary model was a highly technical GPS data model, tracking key indicators across the country’s mines to create a holistic picture of movement and trends in the country. The survey collected never-before-analyzed data from sites, but even initial data quality checks revealed some problems. Thus, the project team implemented two other collection models to supplement the data in the first.
The first round of data collection — the GPS model — proved far too technical for the volatile situation in northern Mali, where formalized data collection could raise suspicions and risk researcher safety. Thus, the second round of data collection in the north was purely textual, consisting of casual conversations with mine-site personnel. The researcher’s observations and anecdotes helped the project team to get even the smallest understanding of trends in this dangerous region. The third data type SSG used was photographs of mine-site activity, implemented to help ensure accuracy and cross-check survey results. Knowing mine size and production can help to improve taxation efficiency and accuracy, but miners may want to diminish their reported production to reduce potential taxes, meaning that mine-site personnel may not accurately report gold production and scale of the mines.
Moreover, the presence of dangerous actors working in and around gold mining may further increase the risk of falsely reported production numbers, as miners might fear antagonizing criminal organizations or violent extremist groups. To cross-reference reported production figures, the project used photographs to document mine-site activity and get a visual understanding of how many people engaged in mining and how much gold they regularly produced. Based on the observable trends demonstrated in the photographs, SSG could better understand the scope of mining activity at the sites.
These three types of data used for SSG — spatial GPS data, textual reporting of conversations, and photographic evidence of daily mine-site activity — represent a data collection mechanism that is conscious of the locality’s limitations. Creative thinking around what qualifies as data and how different data types can complement each other allowed the project team to develop a comprehensive understanding of gold mining despite the difficulties inherent in working in the region. Other creative data types could include video, audio, historical analysis, word-of-mouth, or an infinite number of permutations, variations, and locally-specific models. This is to say that there is no one-size-fits-all data type for locally sensitive data collection — each collection model must be tailored for the situation.
Although these non-numeric data types may not be revolutionary ideas in and of themselves, understanding how development practitioners can use them to inform a fuller and more consistent data set is crucial. In the SSG example, identifying weaknesses in numeric or survey data sets is the first step. Next, one can use a creative, non-numeric data type to “fill in the gaps” where the primary data set might fail. In SSG, this was the casual interviews in the north, helping the project to understand the local situation while maintaining safety. Finally, practitioners can use creative data types to verify the results of the numeric data set. If some of the numbers seem too good to be true, it might be because they are. Cross-referencing outliers or areas of inconsistency using other types of data can ensure quality and reliability in an otherwise suspect data set. In these ways, using creative data in locally-informed ways can increase the quality, accuracy, and reliability of the entire data set.
Reimagining what qualifies as data and how different data types can verify each other is also a good way to move past measuring outputs and towards tracking outcomes. Thinking outside the box in terms of data validity and verification can help to broaden results to include overarching outcome conclusions. For example, in the SSG example, using photographs to understand life at the mine site, including the lack of regulations and safety equipment, can keep the focus on improving the lives of mine-site workers — the ultimate outcome-level objective of the project. This can prevent tunnel vision on gold production and taxation statistics, which were only meant to be the outputs of SSG, not the outcome. Both output- and outcome-level thinking are important to demonstrate project success, but with single-type data sets it is possible to focus too much on the former. As such, data models based in the local context, and using multiple and creative data types in inventive ways, can result in stronger results, clearer pictures, and greater project success.
Posts on the Chemonics blog represent the views of the authors and do not necessarily represent the views of Chemonics.