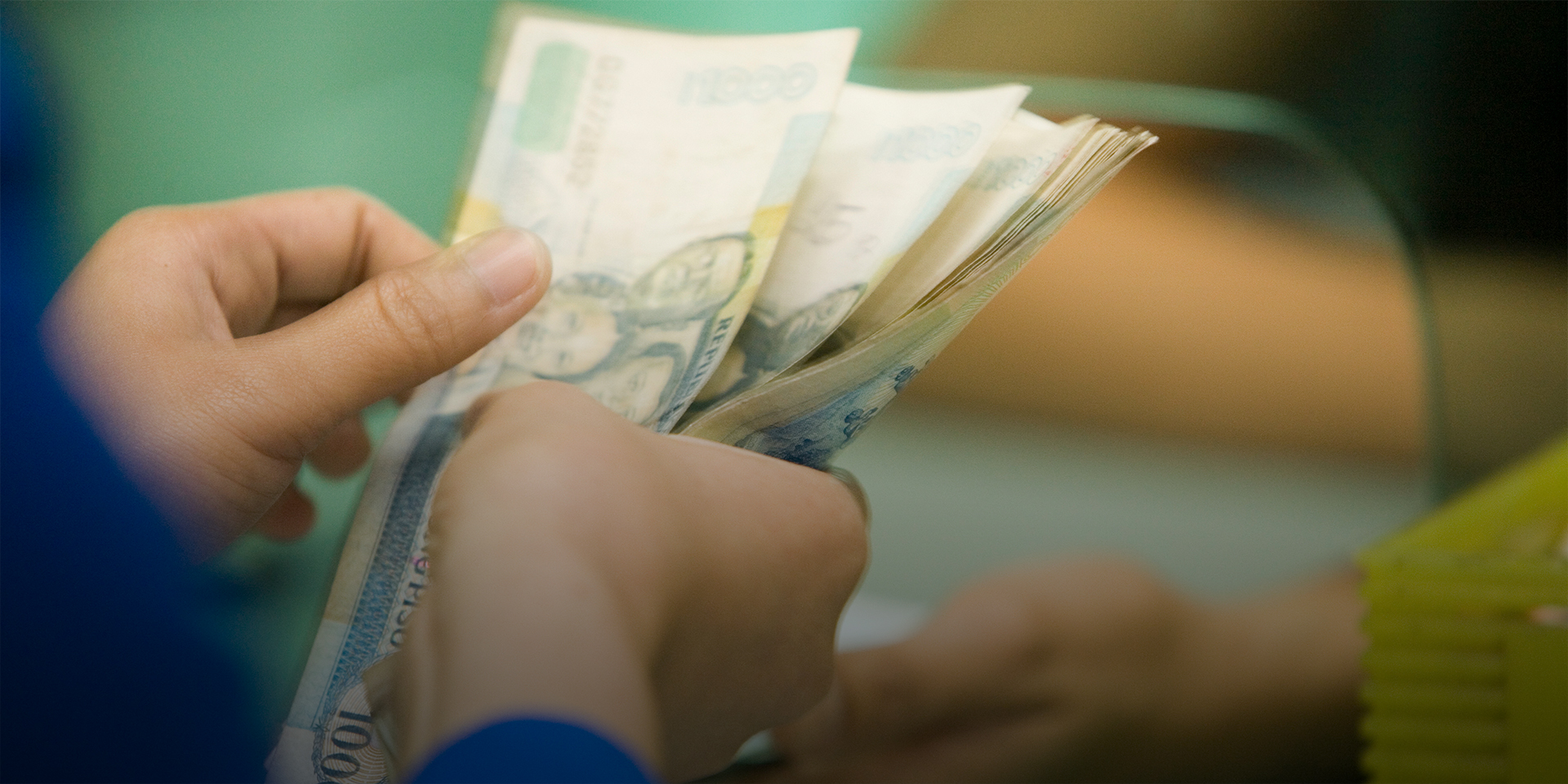
E-Payments Modeling: From Zero to Hero
December 12, 2017 | 4 Minute ReadGetting the right data to measure programmatic success is hard — especially in emerging environments. Jove Tapiador explains how "modeling" might be the solution.
Building working models for evaluation and learning is important to assess the long-term impact and performance of a project. Models can range from conceptual frameworks to algorithms in electronic spreadsheets — or to more sophisticated computer-aided visual simulations. But how do we build a model from scratch when data is sparse to begin with? What methods can be applied to new fields of development practice such as electronic payments? How can we ensure the continued validity of such models over time and harness their predictive power?
Evaluation models are affected not just by availability of data, but by external institutional partners and basic data infrastructure conditions. Therefore, these models should cover both the technical aspects of project evaluation and also include the ways in which external regulatory and programmatic changes influence the monitoring function. The monitoring and evaluation practitioner must be on top of the situation as these conditions create a dynamic and fluid environment wherein the underlying data tracked shifts over time.
These questions are already challenging in countries with existing data and supportive technology infrastructure, but what about an emerging economy with a less sophisticated data infrastructure? The USAID Philippines E-PESO project is addressing these issues within the context of a challenging field of study (electronic payments for financial inclusion) in an emerging economy.
E-Payments and Model Building
Improving financial inclusion through electronic payments (e-payments) has been an evolving field in development practice for the past 13 years. With the explosion of start-up companies focused on financial technology in the last five years, e-payments have further gained global interest. Many of these startups target the unbanked and underbanked, who comprise 2.5 billion of the world’s population. As part of such financial inclusion strategies, understanding electronic payments usage, volume, value, and behavior is critical in developing policies that bring the unbanked and underbanked into the formal financial system and grant access to better savings, loans, and insurance products designed to boost incomes, facilitate entrepreneurship, and protect from life shocks.
Although e-payments data is available for many middle-income and high-income countries because of their existing banking infrastructure, many emerging economies do not have detailed information on e-payments transactions. Much of the existing user data only captures those already banked and is usually stored within banks, without opportunity for aggregation and analysis because of privacy laws or lack of analytical systems.
In the Philippines, E-PESO faces such challenges of identifying and consolidating fragmented, stale, and possibly non-existent data to build a picture of the e-payments landscape in the country. Such data is essential to crafting banking regulations that seek to liberalize the payments industry of the Philippines, while ensuring that benefits of e-payments can be enjoyed even by those who have no formal banking accounts.
At first, E-PESO identified three data sources to get a full picture: Philippine central bank data, bank industry-generated data including industry surveys, and national surveys commissioned by USAID. However, due to challenges in obtaining needed data from the first two sources, E-PESO had to commission a survey firm to generate the required data sets through a nationally representative sample of 1,200 respondents with a margin of error of plus or minus 3 percent and a 95 percent confidence level. E-PESO then used the analytical framework of the Better Than Cash Alliance (BTCA) to analyze payment streams. The results provided E-PESO with the data sets needed for e-payments model building.
Table 1: The payment grid: types of payments by payer and payee
PAYEE |
||||
Person |
Business |
Government |
||
PAYER |
Person |
P2P Remittances, Gifts |
P2B Utilities, Purchases of goods and services, Cellphone load, Loan repayments, School fees |
P2G Taxes, Payment to government utilities, Licenses, Registration fees and other fees |
Business |
B2P Salaries and benefits |
B2B Payment to suppliers, Utilities |
B2G Taxes, fees for licenses and permits, Payment to government utilities |
|
Government |
G2P Conditional Cash Transfers, Salaries, Scholarships, Benefits, Pensions, other Welfare programs |
G2B Supplier Payments, Utility Payments |
G2G National government disbursements to local level, Inter-agency transfers |
Source: Better than Cash Alliance, 2015
Key Drivers of E-Payments
During model building, E-PESO took into consideration demographic and economic secondary, publicly available data to build a dynamic mathematical spreadsheet model to illustrate drivers of e-payments transaction growth. E-PESO tracked multiple factors including population growth, economic growth, e-payments user growth, and some derived data on e-payments usage linked to various payments flows such as person-to-person (P2P), person-to-business (P2B), person-to-government (P2G), etc. Over time, this model has been refined to gain a more nuanced understanding of Philippine e-payment streams.
Using the model, E-PESO was able to identify four key factors affecting the rapid adoption of e-payments in the Philippines: working-age millennial population growth rates; gross domestic product growth rates largely influenced by government, business, and consumer spending; regulatory/policy environment affecting payments practices and industry behavior; and, critically, banking infrastructure interoperability for the National Retail Payment System through automated clearing houses (ACHs) where transactions are cleared and settled. These have significant impact on whether e-payments reach 10 to 20 percent of total retail payments in the Philippines.
Lessons Learned
To overcome the challenges of evaluating the fluid environment of e-payments, E-PESO learned to develop a model that recognizes different socio-economic and policy dimensions. This allows a more holistic understanding on how e-payments adoption can gain traction in the Philippines. As a result, this opens new avenues for model building and prediction that is relevant not just to its host country, but for other projects facing constraints in data for monitoring and evaluation.
In the future, such basic spreadsheet models can evolve into algorithmic models that take advantage of artificial intelligence and big data to automatically track changes in the e-payments environment in near-real time. These can be powerful tools to manage the payments system; generate timely reports needed by regulators for policy decisions; and track macro-economic, demographic, and regulatory changes affecting the payments ecosystem over time.